With very little data, NVIDIA found a way to train AI
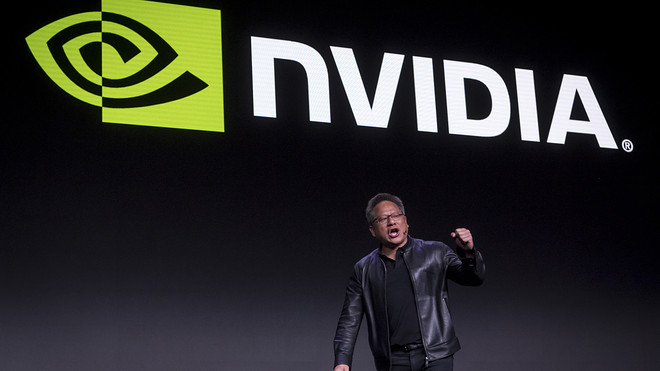
NVIDIA has built up another methodology for preparing generative adversarial networks (GAN) that would one be able to day make them reasonable for a more noteworthy assortment of assignments.
Prior to getting into NVIDIA’s work, it assists with knowing somewhat about how GANs work. Each GAN comprises of two contending neural networks: a generator and a discriminator.
In one where the objective of the calculation is to make new pictures, the last is the thing that inspects a great many example pictures. It at that point utilizes that information to “coach” its counterpart.
To make reliably conceivable outcomes, conventional GANs need some place in the scope of 50,000 to 100,000 preparing pictures. With excessively barely any, they will in general run into an issue called overfitting. In those examples, the discriminator needs more of a base to adequately mentor the generator.
Previously, one way AI scientists have attempted to get around this issue is to utilize a methodology called information increase. Utilizing a picture calculation as an illustration once more, in examples where there isn’t a great deal of material to work with, they would attempt to get around that issue by making “distorted” copies of what is accessible.
Mutilating, for this situation, could mean cropping a picture, rotating it or flipping it. The thought here is that the network never observes a similar identical picture twice.
The issue with that approach is that it would prompt a circumstance in which the GAN would figure out how to mimic those distortions, rather than making something new.
NVIDIA’s new adaptive discriminator augmentation (ADA) approach actually utilizes information expansion yet does so adaptively. Rather than distorting pictures all through the whole preparing measure, it does specifically and barely enough so the GAN dodges overfitting.
The likely result of NVIDIA’s methodology is more important than you may might suspect. Preparing an AI to compose another content based experience game is simple in light of the fact that there’s such a great amount of material for the calculation to work with.
The equivalent isn’t valid for a great deal of different errands analysts could go to GANs for help. For instance, preparing a calculation to recognize an rare neurological brain disorder is troublesome precisely as a result of its rarity. However, a GAN prepared with NVIDIA’s ADA approach could get around that issue.
If that wasn’t already enough, specialists and scientists could share their discoveries all the more effectively since they’re working from a base of pictures made by an AI, not patients in reality. NVIDIA will share more data about its new ADA approach at the forthcoming NeurIPS meeting, which begins on December sixth.